- SC-Block: Supervised Contrastive Blocking within Entity Resolution Pipelines
Abstract: Millions of websites use the schema.org vocabulary to annotate structured data describing products, local businesses, or events within their HTML pages. Integrating schema.org data from the Semantic Web poses distinct requirements to entity resolution methods: (1) the methods must scale to millions of entity descriptions and (2) the methods must be able to deal with the heterogeneity that results from a large number of data sources. In order to scale to numerous entity descriptions, entity resolution methods combine a blocker for candidate pair selection and a matcher for the fine-grained comparison of the pairs in the candidate set. This paper introduces SC-Block, a blocking method that uses supervised contrastive learning to cluster entity descriptions in an embedding space. The embedding enables SC-Block to generate small candidate sets even for use cases that involve a large number of unique tokens within entity descriptions. To measure the effectiveness of blocking methods for Semantic Web use cases, we present a new benchmark, WDC-Block. WDC-Block requires blocking product offers from 3,259 e-shops that use the schema.org vocabulary. The benchmark has a maximum Cartesian product of 200 billion pairs of offers and a vocabulary size of 7 million unique tokens. Our experiments using WDC-Block and other blocking benchmarks demonstrate that SC-Block produces candidate sets that are on average 50% smaller than the candidate sets generated by competing blocking methods. Entity resolution pipelines that combine SC-Block with state-of-the-art matchers finish 1.5 to 4 times faster than pipelines using other blockers, without any loss in F1 score. - Do Similar Entities have Similar Embeddings?
Abstract: Knowledge graph embedding models (KGEMs) developed for link prediction learn vector representations for entities in a knowledge graph, known as embeddings. A common tacit assumption is the KGE entity similarity assumption, which states that these KGEMs retain the graph’s structure within their embedding space, i.e., position similar entities within the graph close to one another. This desirable property make KGEMs widely used in downstream tasks such as recommender systems or drug repurposing. Yet, the relation of entity similarity and similarity in the embedding space has rarely been formally evaluated. Typically, KGEMs are assessed based on their sole link prediction capabilities, using ranked-based metrics such as Hits@K or Mean Rank. This paper challenges the prevailing assumption that entity similarity in the graph is inherently mirrored in the embedding space. Therefore, we conduct extensive experiments to measure the capability of KGEMs to cluster similar entities together, and investigate the nature of the underlying factors. Moreover, we study if different KGEMs expose a different notion of similarity. Datasets, pre-trained embeddings and code are available at: https://github.com/nicolas-hbt/similar-embeddings/. - Column Property Annotation using Large Language Models
Abstract: Column property annotation (CPA), also known as column relationship prediction, is the task of predicting the semantic relationship between two columns in a table given a set of candidate relationships. CPA annotations are used in downstream tasks such as data search, data integration, or knowledge graph enrichment. This paper explores the usage of generative large language models (LLMs) for the CPA task. We experiment with different zero-shot prompts for the CPA task which we evaluate using GPT-3.5, GPT-4, and the open-source model SOLAR. We find GPT-3.5 to be quite sensitive to variations of the prompt, while GPT-4 reaches a high performance independent of the variation of the prompt. We further explore the scenario where training data for the CPA task is available and can be used for selecting demonstrations or fine-tuning the model. We show that a fine-tuned GPT-3.5 model outperforms a RoBERTa model that was fine-tuned on the same data by 11% in F1. Comparing in-context learning via demonstrations and fine-tuning shows that the fine-tuned GPT-3.5 performs 9% F1 better than the same model given demonstrations. The fine-tuned GPT-3.5 model also outperforms zero-shot GPT-4 by around 2% F1 for the dataset on which is was fine-tuned, while not generalizing to tasks that require a different vocabulary.
Three papers accepted for ESWC 2024
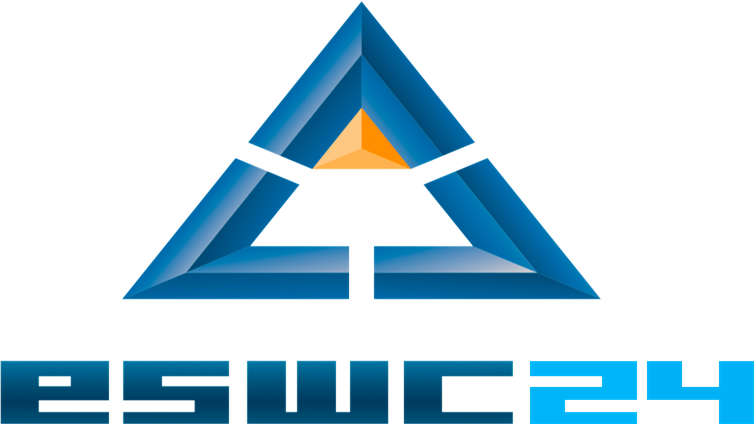