Digitalization of the Tax Function
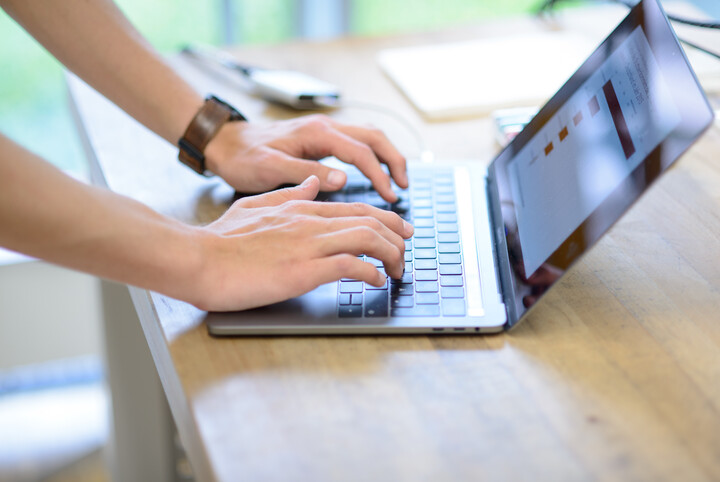
Author(s):
Christoph Spengel, Alexander Maedche, Sven Scheu, Sarah Winter
Cooperation Partners:
Institute of Information Systems and Marketing (IISM) at KIT, EnBW AG
Description:
Tax departments are facing new regulatory requirements to provide an increasing amount of information to tax authorities. Thus, being able to process structured and unstructured tax data in a timely manner and improve decision making processes is essential.
We collaborate with a major German energy supplier to develop new concepts for the digital transformation of the tax function. The project focuses on optimizing processes in the tax department through digital means and enhancing existing tax information systems and intragroup information processing. The project also aims at developing data-driven intelligent assistant functions to support decision-making processes using Process Mining, Business Intelligence, and more generally spoken Data Science.
Driving forward while looking back: Employee responses to error amidst when monitored by emergent technologies
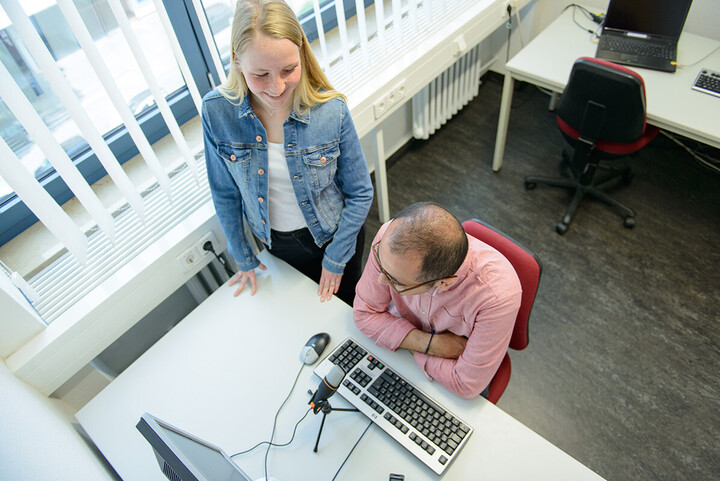
Author(s):
Rajiv Sabherwal, Hartmut Höhle, Tobias Nisius, Florian Pethig, Fareed Zandkarimi
Cooperation Partners:
Aalberts Material Technology GmbH
Description:
In this project we are examining the effect of reclamations on several behaviors of production staff. Using process mining allows us to measure production-level behavior of workers. These measures are collected over a three-year period and are planned to be analyzed using a difference-in-difference (DID) design. The expected results should broaden our understanding of employee responses to error.
We are looking into core-production data of a very large company. Applying statistical methods on production-level data, combined with the application of process mining, is one major contribution of our work. Also, the expected outcomes related to the research problem, i.e., employee responses to reclamations, provide practitioners new insights on making effective decisions before and after an error is reported.
During the data collection phase, we collected 1.4 million production cases via access to the company’s enterprise resource planning (ERP) system. In order to apply process mining, 500+ lines of SQL code were developed to transform the data into processable event logs. Also, a Java program was written to collect appropriate data for the DID analysis. The aggregated dataset is currently analyzed using Stata.
Process Mining meets Procurement: Application of Process Mining Methods to Measure Inefficiencies in Procurement Processes
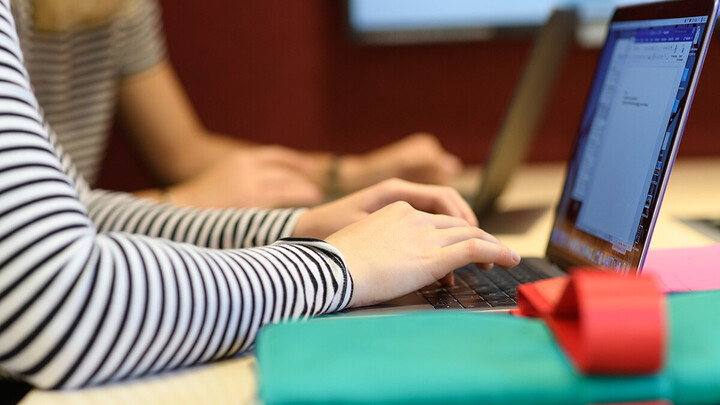
Author(s):
Prof. Dr. Christoph Bode, Prof. Dr. Hartmut Höhle, Jun.-Prof. Dr. Jana-Rebecca Rehse, Jonas Ronellenfitsch, Fareed Zandkarimi
Cooperation Partners:
Schott AG, Hilti AG, HeidelbergCement AG und Heidelberger Druckmaschinen AG
Description:
Procurement processes, such as purchase-to-pay (P2P), are of central importance to companies. Problems and inefficiencies in these processes can not only cause delays in production, but also lead to significant additional costs. However, limited research has been conducted on these processes, particularly at the operational level, meaning that it is not known what inefficiencies occur in the actual execution of the processes, where they come from, and how they can be prevented if necessary.
To address this question, an interdisciplinary research team of process mining and procurement experts is investigating how methods of process mining, i.e., the analysis of process execution data, can be applied to identify, measure, explain, and ideally eliminate inefficiencies in procurement processes. To this end, data from P2P processes of three large industrial companies are analyzed and examined for common patterns. Based on this research, new key performance indicators (KPIs) are developed that precisely quantify the inefficiency of a P2P process. These KPIs can now be used, for example, to identify temporal patterns in process inefficiency or to highlight the impact of an external influence, such as the Corona pandemic, on procurement processes.