Variation in review writing behavior across time-of-the-day, week day, season, weather conditions, a city’s life-quality and other factors
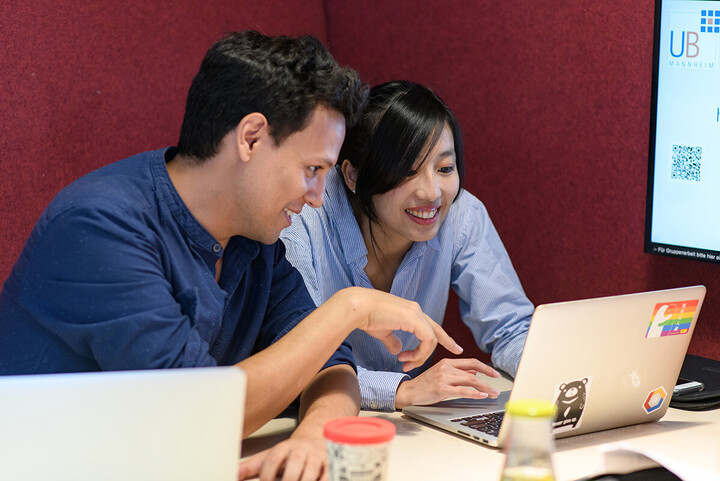
Author(s):
Andreas Bayerl, Florian Stahl, Jacob Goldenberg
Cooperation Partners:
IDC Israel, Kununu.de
Description:
We analyze a data set of in total more than 10 Mio online reviews from two different platforms collecting User-Generated Content to find differences in review writing behavior across time-of-the-day, week day, season, weather conditions, a city’s life quality and other factors. Online reviews are meant to be an unbiased source of information. We will show how in fact, they can be influenced by externalities that have nothing or very little to do with the reviewed instance. By doing so we will follow a suggestion by Miller (2011) from his Science article saying that the power of the Internet and unstructured data can help take research to the next level. Methods employed during this project are among others, web scraping to acquire data, Natural Language Processing (NLP) to analyze data and Machine Learning to develop a model predicting review scores based on externalities.
The Impact of the Offered Product Mix on the Acquisition, Usage and Retention of Information Goods
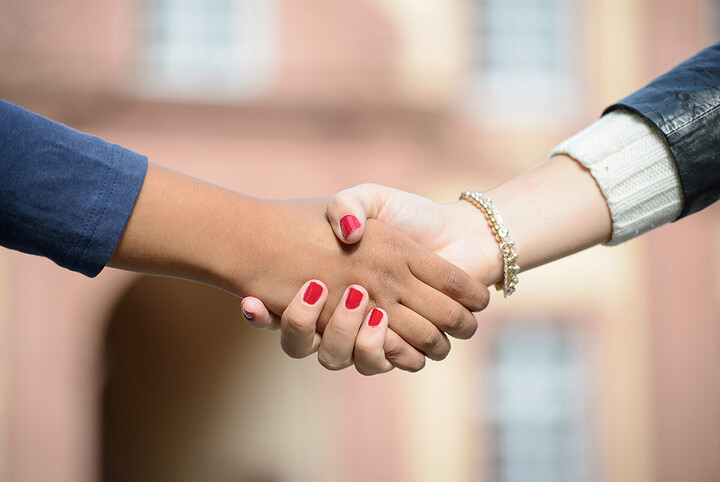
Author(s):
Daniela Schmitt, Raghuram Iyengar, Florian Stahl
Cooperation Partners:
Wharton School (Philadelphia)
Description:
A primary challenge for information goods providers (e.g., news websites) is maintaining a balance between their free users and paid subscribers by determining which of the former are likely to convert to the latter and when. We address questions of what kinds of content do users consume and how much do the inter-session consumption dynamics impact if/
Theoretically, our results help understand different users' responses in usage behavior to product mix changes both in the short and in the long run. From a managerial perspective, our results provide actionable implications for firms that employ the freemium business model (e.g. newspaper websites, video or music streaming services) on how a change in their product mix can impact the user experience and behavior.