The signal-to-noise ratio hypothesis: A computational perspective on general intelligence
Anna-Lena Schubert, 2 July 2025, 14:00
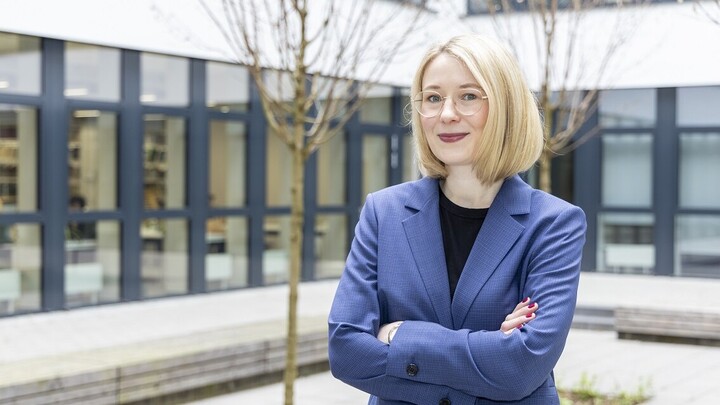
The positive manifold underlying general intelligence suggests a common cause—a feature of the cognitive system that influences performance globally, irrespective of the type of information processed or the specific task. In this talk, I will argue that the signal-to-noise ratio (SNR) of cognitive processing serves as such a global determinant of individual differences. SNR governs the fidelity of information processing across all cognitive operations. A stable, system-wide SNR would therefore be expected to impact performance across diverse cognitive tasks, making it a strong candidate for explaining the general factor of intelligence (g). In computational models of cognition, individual differences in performance typically arise from variations in model parameters. A global characteristic like SNR would be reflected in parameters common to models of cognitive activities that contribute to the positive manifold. By comparing models of simple decisions, sensory discrimination, categorization, decision-making, learning, and working memory, a shared feature emerges: a parameter linked to SNR, manifesting either as variable signal strength against a fixed noise baseline or as a noise parameter varying across individuals or tasks. I will present initial evidence supporting the SNR hypothesis of intelligence, highlighting findings from evidence accumulation models of decision-making and models of working memory, where SNR parameters correlate significantly with fluid intelligence. Furthermore, I will demonstrate how a neurocognitive computational modeling approach can address the identifiability challenges in computational models, enabling the estimation of signal and noise as distinct, interpretable parameters. This framework offers a promising path to deepen our understanding of the cognitive and computational basis of intelligence.