Thilo Dieing, Chair of Artificial Intelligence: TransforMA and NEST-BW
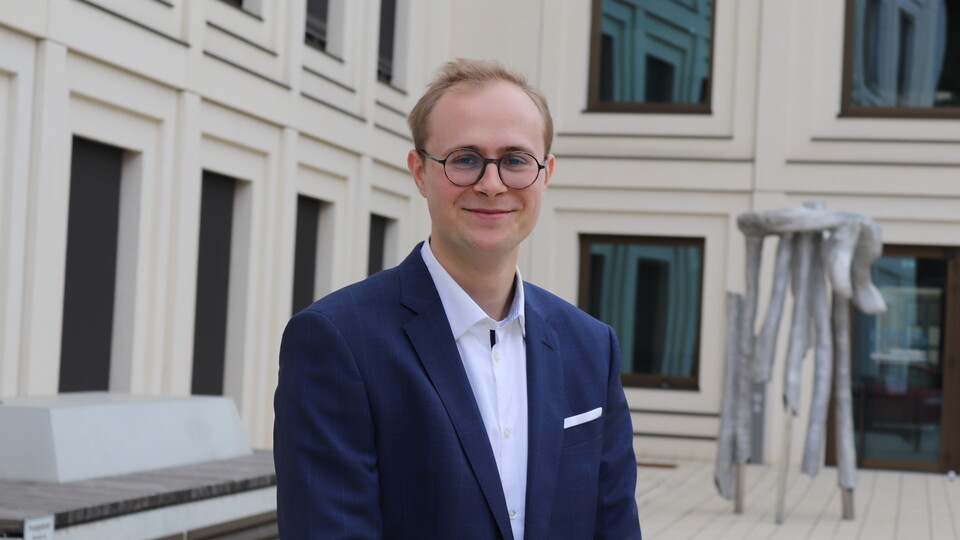
What is your current research topic?
My first research topic at the AI group was in the TransforMA project. Here I was part of a six-person student team, as part of the Mannheim Master in Data Science, that wanted to take a closer look at language analysis. After the successful TransforMA project, in which we built an audio-based, multilingual recipe chatbot called Foodle, I am now part of the AI team in the NEST-BW project. The aim is to use suitable AI measures to optimize the study orientation for prospective students in order to enable a better match between first-year students and their study program. This should lead to a lower dropout rate. As part of this, we have, for example, developed a chatbot based on generative AI that can answer all the important questions prospective students have about the orientation phase and the possible courses of study. What is particularly important to us here is the safe use of such tools to avoid a negative impact.
For those who have not yet delved deeply into the topic of data science: How would you explain to a child what you are working on?
Imagine you want to know what kind of degree program you might want to pursue later on. Our chatbot, a computer that can talk to you as well as a human, can help with this question. But the chatbot is even smarter because it has a huge amount of knowledge about different degree programs. And the best thing about it is that the chatbot not only knows a lot, but it can also quickly look up what exactly matches your interests and talents.
So if you have a question, for example, “What can I study if I like animals?” the chatbot not only gives you an answer, but searches through a huge collection of information to find what suits you best. It helps you understand what courses are available, what you can learn and where you can study. It's like a clever friend who helps you find your way around so that you can later find the course of study that suits you.
Everyone is talking about data science – how would you describe the importance of the topic for yourself in three words?
Decoding our world
What points of contact does your work have with data science? Which methods do you already use and which would be interesting for you in the future?
Data science is a central theme in every aspect of our work and is therefore indispensable. We are currently focusing on NLP (Natural Language Processing) methods, optimizing open-source large language models (LLM) and establishing uniform data. In the future, we plan to continue using self-trained classification models to further improve existing solutions. I would also be interested in incorporating additional fields, such as speech and image recognition, to enable users to have real conversations with a chatbot, for example, and thus make the user experience even more personal.
How valuable is data science for your work? Would your research even be possible without data science?
Without data science, our work would be completely impossible. It forms the foundation of all our research and development. In particular, data science plays a central role in the area of AI-supported study orientation and the development of chatbots. By analyzing large amounts of data, we can create personalized recommendations for fields of study based on students' individual interests and abilities. With the help of machine learning algorithms, we can continuously improve and adapt these models to make more accurate predictions.
Another essential part of our work is the chatbot, which uses natural language processing and other data science methods to respond to users' needs. Data science enables us to analyze large text and conversation data to train the chatbot to provide increasingly relevant answers, thus enabling effective student advising. The ability to analyze, interpret and transform data into usable information is essential to successfully develop and operate these systems. Without these data-driven approaches, it would not be possible to create such intelligent systems that can respond so individually and precisely to the needs of students.
What development opportunities do you see for the topic of data science in relation to your field?
Since much of what we are currently working on is actively being researched, we are also seeing a great deal of development. Models are improving almost daily/