Jovita Lukasik, Data abnd Web Science Group: Machine Learning (August 2022)
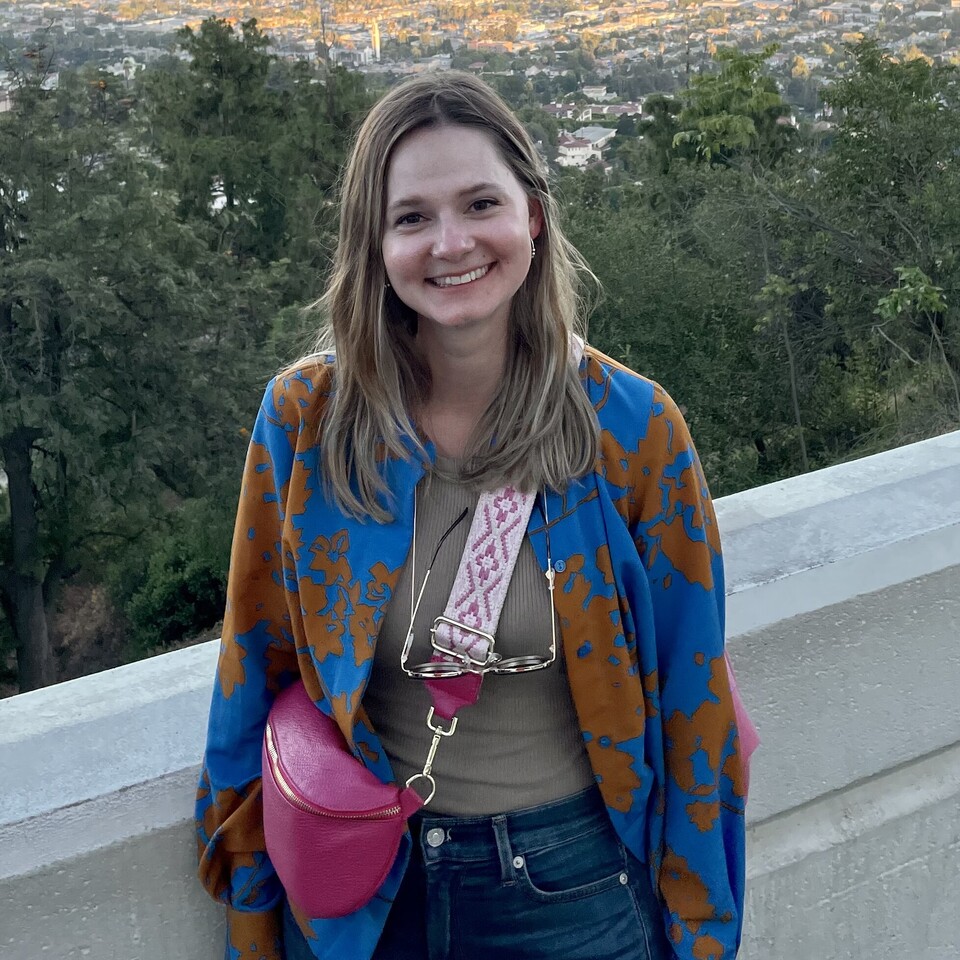
What is your current research topic?
My current research topic is in the area of “Neural Architecture Search” (NAS), based on the BMBF project “Deep Topology Learning”. NAS in general is the search for well-performing architectures (neural networks). Beginnings in this area were based on training and evaluating several thousand architectures, which is extremely time consuming and expensive. Our group in the project aimed to make the search more efficient by first developing embeddings of architectures with the idea that this embedding can be used by a regression model to make predictions about the performance of the generated network.
For those who have not yet delved deeply into the topic of Data Science: How would you explain to a child what you are working on?
My field is about making it easier and automate the process for the computer to learn certain things. Let's say we have many different pictures with street signs on them. Now you want the computer to tell you exactly what kind of road sign is on each picture. If we are all ever sitting in self-driving cars, we would like the car to know exactly what sign is there. Until now, you have to use a lot expert knowledge to program the computer to recognize the street signs correctly. Now we just want to use as little human expert knowledge as possible and automate the computer programming process.
Everyone talks about Data Science – how would you describe the importance of the topic for yourself in three words?
necessary, machine learning, analysis
What points of contact with Data Science does your work have? Which methods do you already use, and which would be interesting for you in the future?
The NAS area is based on various machine learning approaches and thus on data science. Without Machine Learning (ML) neural networks would not exist and therefore NAS would not exist. Also, python is our daily bread. We are searching for neural networks with ML tools. For the future, I would find it interesting if more data from my field were accessible to everybody. Trained networks as well as the exact approaches used to find networks to push the field faster and further.
How high is the value of Data Science for your work? Would your research even be possible without Data Science?
No. My research would also not exist. As I said, all my work is based on MLs tool with the goal to find and design neural networks.
What development opportunities do you see for the topic of Data Science in relation to your field?
Ideally, we will have reached the point where, without a lot of expert knowledge, we are able to find neural networks that perform very well and are ideally interdisciplinary; networks for Computer Vision, Natural Language Processing and much more. But this requires a lot of research as well as a lot of transparency about the NAS methodologies.