Tree-based Machine Learning for Dynamic Datastreams
Research Problem
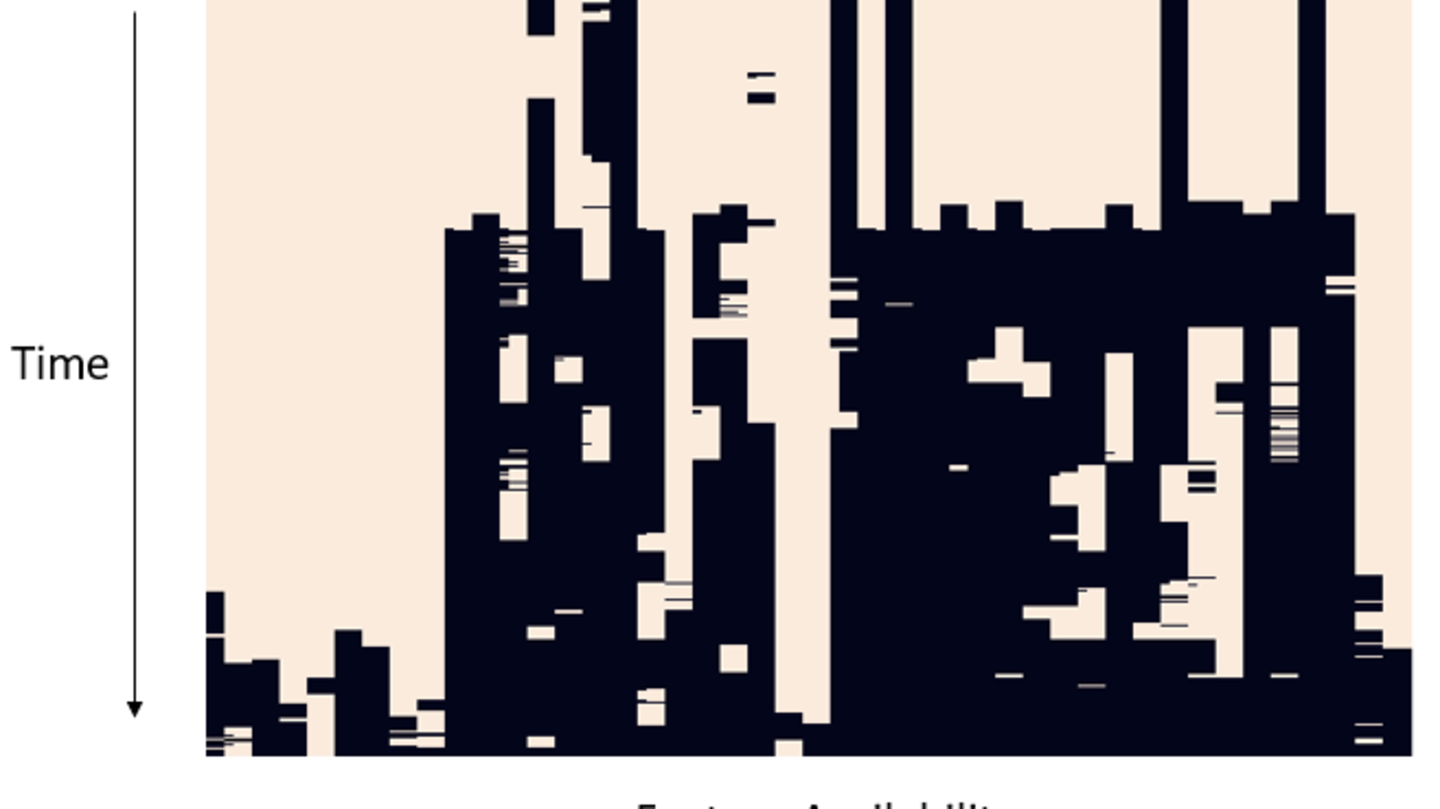
The ability to handle more and more data has led to an ever-increasing amount of available data, which in turn brought the demand to process and handle this data on the fly. Online learning is a class of machine learning algorithms aiming to solve this problem, hereby training instances are processed sequentially, and a model is learned on the fly, as opposed to offline learning, where a fixed set of instances and features are given, and the learning algorithm derives a model based on this fixed input. However, the assumption of online learning that the feature space remains fixed and only the instance space is continuously growing does not hold in all applications. For example, in a crowd-sensing scenario, new sensors will be added over time, if users join the sensing effort, while others may vanish due to users leaving the crowdsensing platform or sensors breaking. This problem, where the feature space may grow, due to new features that emerge, or shrink, because of vanishing features, was introduced as varying feature spaces. The focus of this work is to develop Decision Tree-based approaches to deal with the dynamics of the data streams in various scenarios.
Contact
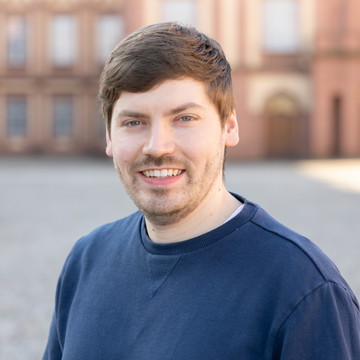
Christian Schreckenberger
Institute for Enterprise Systems
L15, 1–6 – Room 416
68161 Mannheim
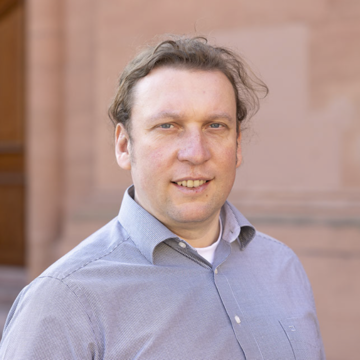
Prof. Dr. Christian Bartelt
Institut für Enterprise Systems
L 15, 1–6 – Room 417
68161 Mannheim
E-mail: christian.bartelt uni-mannheim.de
Web: www.uni-mannheim.de/en/ines/about-us/researchers/dr-christian-bartelt