Christoph Kern, Lehrstuhl für Social Data Science und sozialwissenschaftliche Methodenlehre: Fairness beim maschinellen Lernen (Juli 2022)
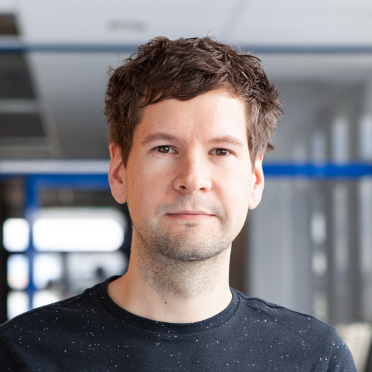
What is your current research topic?
Most of my recent work focuses on fairness in machine learning. A current example is the project “Fairness in Automated Decision-Making – Fair ADM” (jointly with Frauke Kreuter, LMU Munich, and Ruben Bach, University of Mannheim), in which we study the implications of algorithmic profiling of job seekers. This includes building profiling models with German administrative data, and conducting “fairness audits”. That is, we are addressing questions such as: What are the downstream effects of different modeling decisions? Do we observe differences in model performance for certain (protected) subgroups? How do certain types of error rates compare between, e.g., German and non-German jobseekers?
For those who have not yet delved deeply into the topic of Data Science: How would you explain to a child what you are working on?
In my research field, many people are trying to ensure that computer programs make good decisions. Imagine, for example, a computer decides whether you get some extra help in a difficult class in school. Of course, you wouldn’t want the computer to decide based on your skin tone or the colour of your hair. Instead, we want to make sure that those who need extra support are correctly assigned by the computer and receive help, independent of attributes that should not matter for such decisions.
Everyone talks about Data Science – how would you describe the importance of the topic for yourself in three words?
statistics + computer science + ambiguity
What points of contact with Data Science does your work have? Which methods do you already use, and which would be interesting for you in the future?
We use various machine learning methods in the Fair ADM project to build algorithmic profiling models. An interesting aspect is that modern data science tools can be paired with “old” or traditional data, and oftentimes we see that this can improve things, e.g., with respect to prediction performance. However, the question is whether this still holds true once we take a closer look into subgroup performance, or consider interpretability. In the future, I think it will be interesting to (empirically) evaluate the use of data science methods to improve the allocation of, e.g., labor market programs or other support measures for unemployed individuals, with respect to both performance and fairness.
How high is the value of Data Science for your work? Would your research even be possible without Data Science?
The increasing use of algorithmic decision-making (ADM) in various contexts is inevitably tied to advances in data science methodology. Of course, ADM systems can be implemented in different ways and may draw on various types of statistical models, but the advent of powerful machine learning methods clearly fueled the use of prediction approaches to support decision-making. Since my work is studying social implications of ADM, it is in a sense quite dependent on historical progress in data science and statistics.
What development opportunities do you see for the topic of Data Science in relation to your field?
Having a background in social science and survey methodology, I’m hoping for more interdisciplinary collaborations with a strong focus on data quality in data science contexts. Technical progress is great, but it is also of great importance to develop a joint understanding of the implications of using insufficient training data. I think a lot of work can be done on improving generalizability and representation to promote a safe and reliable usage of data science tools.