Paper accepted at EMNLP Findings 2024
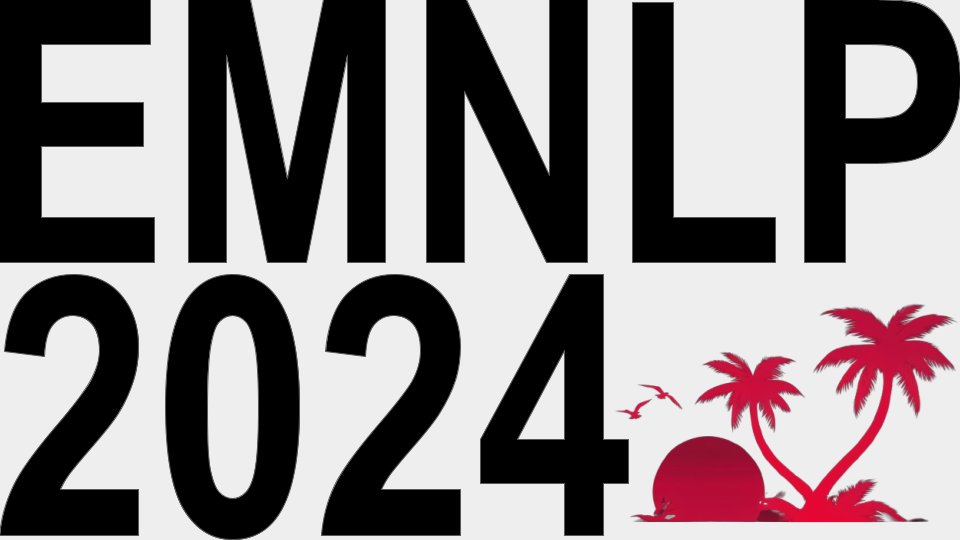
The paper “Train Once, Use Flexibly: A Modular Framework for Multi-Aspect Neural News Recommendation” by Andreea Iana, Goran Glavaš, and Heiko Paulheim has been accepted at the Findings of the 2024 Conference on Empirical Methods in Natural Language Processing.
Abstract:
Recent neural news recommenders (NNRs) extend content-based recommendation (1) by aligning additional aspects (e.g., topic, sentiment) between candidate news and user history or (2) by diversifying recommendations w.r.t. these aspects. This customization is achieved by ``hardcoding`` additional constraints into the NNR's architecture and/