Paper accepted at ISWC 2021
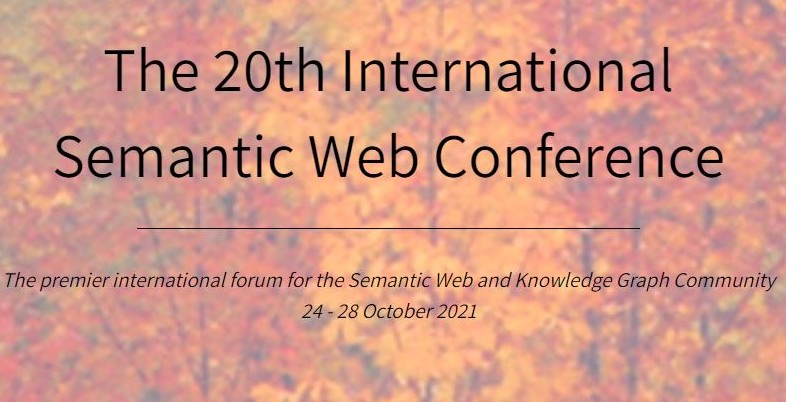
The paper “Graph-boosted Active Learning for Multi-Source Entity Resolution” by Anna Primpeli and Christian Bizer was accepted at the International Semantic Web Conference, which had an acceptance rate of 18% this year.
Abstract
Supervised entity resolution methods rely on labeled record pairs for learning matching patterns between two or more data sources. Active learning minimizes the labeling effort by selecting informative pairs for labeling. Most active learning methods for entity resolution proposed so far focus on the two-source matching setting and use committee-based or margin-based strategies for picking informative pairs for labeling. In this paper, we propose ALMSER, a graph-boosted active learning method for multi-source entity resolution. To the best of our knowledge, ALMSER is the first active learning-based entity resolution method that is especially tailored to the multi-source setting. ALMSER exploits the rich correspondence graph that exists in multi-source settings for selecting informative record pairs. In addition, the correspondence graph is used to derive complementary training data. We evaluate our method using five different multi-source matching tasks having different profiling characteristics. The experimental evaluation shows that ALMSER outperforms active learning methods using margin-based and committee-based query strategies, in terms of F1 score on all tasks.
Preprint Version of the Paper
Anna Primpeli, Christian Bizer: Graph-boosted Active Learning for Multi-Source Entity Resolution.
More information on ISWC 2021 here