Best Paper Award at iiWAS 2024
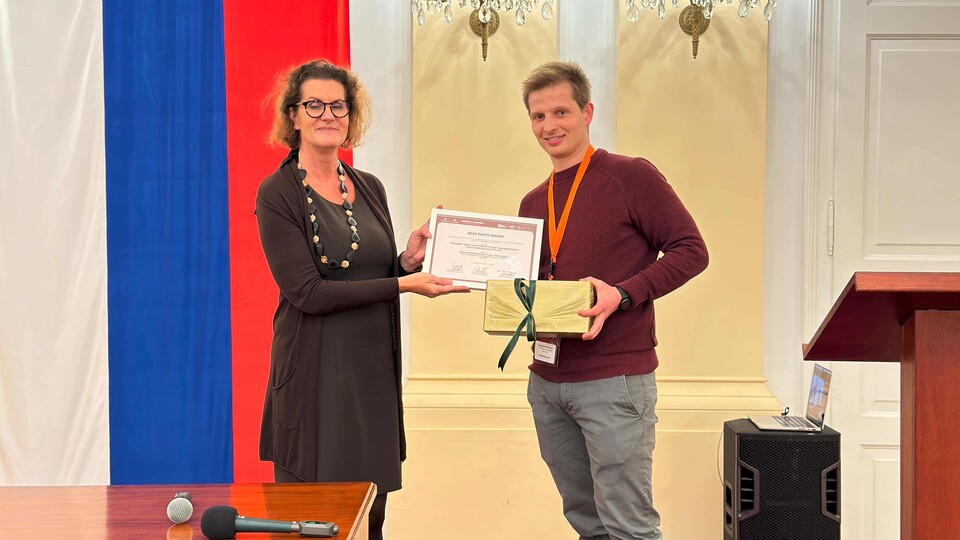
The abstract and a link to the paper can be found below:
ExtractGPT: Exploring the Potential of Large Language Models for Product Attribute Value Extraction
In order to facilitate features such as faceted product search and product comparison, e-commerce platforms require accurately structured product data, including precise attribute/
Please find the slides for the talk at iiWAS2024 here: Slides.