Kartellrechtskonforme KI (KarekoKI)
Project Partners
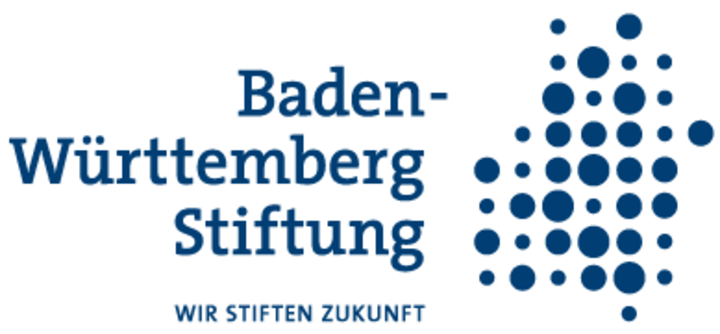
- University of Mannheim, Chair of Public Law, Regulatory Law and Tax Law,
- University of Mannheim, Chair of Data Science
Key Idea
Automatic price fixing increasingly takes place on online platforms such as Amazon. Here, Artificial Intelligence (AI) systems are used more and more to monitor the prices of competitors and set apply that to their platforms prices. While such market observation and reaction is immanent to competition and therefore permissible under cartel law (so-called “parallel behaviour”), an agreement on prices (collusion) is prohibited by competition law.
Recent research literature on artificial intelligence suggests that AIs are generally capable of developing agreement strategies, even if they are not explicitly programmed to do so. This raises questions on how to deal with this potential for (illegal) price fixing by AIs.
Within the framework of this project it is to be investigated (1) which pricing algorithms has the potential to independently develop cooperation strategies and how it can be implemented on the basis of the current the legal situation. Based on this, legal and technical solutions are designed in order to (2) automatically discover such cooperations. Finally, a legal framework and strategies will be developed, on how (3) AI-based price fixing can be technically prevented.
Funding
The project is funded by the German Baden-Württemberg Stiftung (BW-Stiftung) under the funding scheme responsible artificial intelligence with a funding amount of 383.000 €.
Publications
- Michael Schlechtinger, Damaris Kosack, Heiko Paulheim, Thomas Fetzer: Winning at Any Cost – Infringing the Cartel Prohibition With Reinforcement Learning. 19th International Conference on Practical Applications of Agents and Multi-Agent Systems.
Duration
2020–2023
Personnel at University of Mannheim
Publications
2024
- Schlechtinger, M. (2024). Investigating, predicting, and mitigating collusive behavior in deep reinforcement learning-based pricing AIs. Dissertation. Mannheim.
- Schlechtinger, M., Kosack, D., Krause, F. and Paulheim, H. (2024). By fair means or foul: Quantifying collusion in a market simulation with deep reinforcement learning. In , Proceedings of the Thirty-Third International Joint Conference on Artificial Intelligence, IJCAI-24 : main track (S. 485–493). , International Joint Conferences on Artificial Intelligence Organization: Darmstadt ; Vienna.
2023
- Schlechtinger, M., Kosack, D., Paulheim, H., Fetzer, T. and Krause, F. (2023). The price of algorithmic pricing: Investigating collusion in a market simulation with AI agents. In , AAMAS '23: Proceedings of the 2023 International Conference on Autonomous Agents and Multiagent Systems (S. 2748-2750). , International Foundation for Autonomous Agents and Multiagent Systems: Richland, SC.
2021
- Schlechtinger, M., Kosack, D., Paulheim, H. and Fetzer, T. (2021). How algorithms work and play together. Concurrences : Revue des Droits de la Concurrence, 2021, 19–23.
- Schlechtinger, M., Kosack, D., Paulheim, H. and Fetzer, T. (2021). Winning at any cost – infringing the cartel prohibition with reinforcement learning. In , Advances in Practical Applications of Agents, Multi-Agent Systems, and Social Good : The PAAMS collection, 19th international conference, PAAMS 2021, Salamanca, Spain, October 6–8, 2021, proceedings (S. 255–266). Lecture Notes in Computer Science, Springer: Berlin [u. a.].