ENGAGE.EU Learning Analytics: Awarded Papers
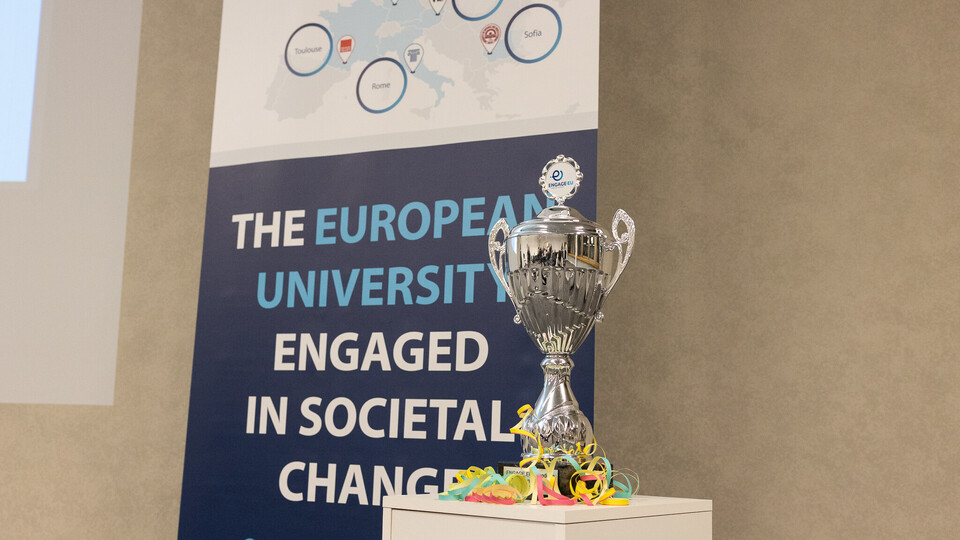
The aim is to develop innovative learning analytics tools to analyze and predict the learning progress of ENGAGE.EU learners. The results will contribute to the development of courses and trainings and can be published in scientific publications.
Among others, two studies by the Mannheim researcher Lea Cohausz (Chair of Artificial Intelligence), which appeared in the publications of the Educational Data Mining Conference in 2022 and 2023, serve as a basis. Both essays were awarded the Best Paper Award.
The first paper of 2022 entitled “Towards real interpretability of student success prediction combining methods of XAI and social science” received the Best Student Short Paper Award. Machine learning is now frequently used to predict academic success or dropout. Apart from the probability of dropping out, the reasons for it are also interesting. However, one disadvantage of Machine Learning methods is that they are often not transparent – one cannot see from the resulting models why a method makes a decision. Therefore, in this paper, a model was developed that makes it possible to depict the interrelationships of variables that have an effect on dropout.
With the paper “Investigating the Importance of Demographic Features for EDM-Predictions” in collaboration with Andrej Tschalzev, Christian Bartelt and Heiner Stuckenschmidt, Lea Cohausz received the Best Student Paper Award. In this paper, they investigated to what extent demographic data play a role for predictions in the Educational Data Mining domain. They are often used in models, although it can lead to situations where people are only admitted to a course of study based on a demographic characteristic (for example gender). In the paper it is shown that demographic variables do not lead to an increase in the performance of the models, but nevertheless lead to major problems in terms of fairness. Accordingly, it is recommended not to use these variables – but this still does not guarantee fairness with respect to these characteristics.
The paper was published on the Homepage of the International Educational Data Mining Society.