WDC Product Data Corpus and Gold Standard for Large-Scale Product Matching Version 2.0 released
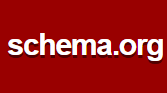
Motivation:
The research focus in the field of entity resolution (aka link discovery or duplicate detection) is moving from traditional symbolic matching methods to embeddings and deep neural network based matching. A problem with evaluating deep learning based matchers is that they are rather training data hungry and that the benchmark datasets that are traditionally used for comparing matching methods are often too small to properly evaluate this new family of methods. Another problem with existing benchmark datasets is that they are mostly based on data from a small set of data sources and thus do not properly reflect the heterogeneity that is found in large-scale integration scenarios. The WDC gold standard and training sets for large-scale product matching tackle both challenges by being derived from a large product data corpus originating from many websites which annotate product descriptions using the schema.org vocabulary. We have performed a set of baseline experiments in order to showcase the suitability of the WDC product corpus as training data as well as the difficulty of the gold standard. The experiments show that deep learning based matchers reach an F1 of 0.90 using the xlarge training set and outperform traditional symbolic matchers by a margin of 16% in F1.
Background:
Many e-shops markup products and offers in their HTML pages using the schema.org vocabulary. In recent years, the e-shops have also started to annotate product identifiers such as gtin8, gtin13, gtin14, mpn and sku. These identifiers allow offers for the same product from different e-shops to be grouped into clusters and can thus be used as supervision for training product matching methods.
The Web Data Commons project regularly extracts schema.org annotations from the Common Crawl, a large public web corpus. November 2017 version of the WDC schema.org data set contains 365 million offers. The WDC product data corpus was derived from this dataset using a cleansing workflow which ignores offers on list pages and only keeps offers annotating interpretable product identifiers. The different steps of the cleansing workflow are described in [PrimpeliPeetersBizer2019].
We think that the creation of the WDC product data corpus nicely demonstrates the utility of the Semantic Web. Without the website owners putting semantic annotations into their HTML pages it would have been much harder, if not impossible, to extract product offers from 79 thousand e-shops and we would likely not have dared to approach this task.
More Information:
More information about the WDC Product Data Corpus and Gold Standard for Large-scale Product Matching is found on the WDC website which also offers both artefacts for public download.